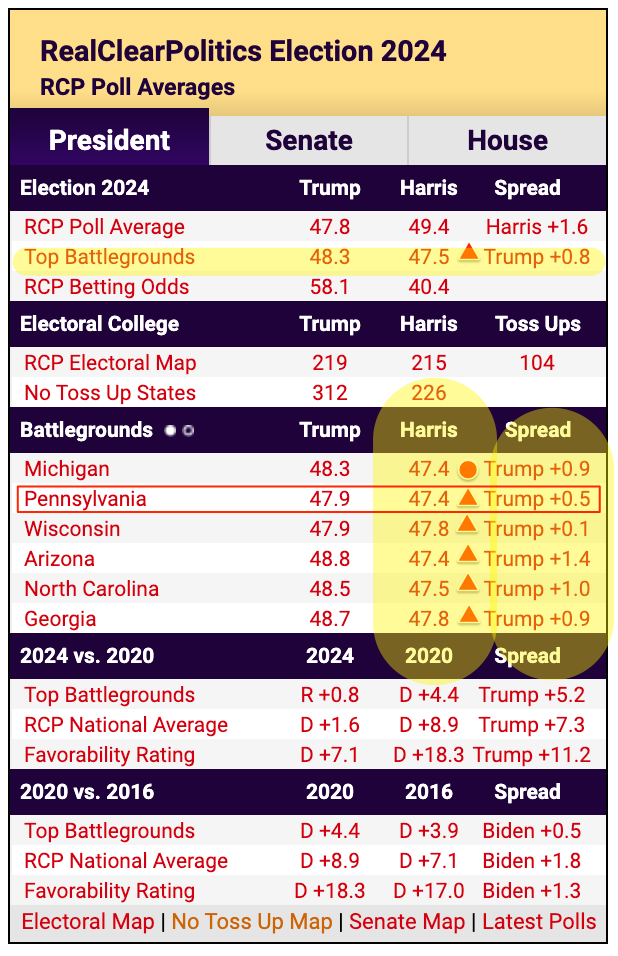
2:00PM Water Cooler 10/23/2024
~ Today's Water Cooler: Politics, Syndemics; 2024 Opinion-havers opine, two weeks out; Trump media blitz (Rogan; Carlson); Kamala and Biden's decline (her view vs. the donor's view); Boeing satellite explodes; Ortberg seeks culture change ~
By Lambert Strether of Corrente.
Bird Song of the Day
I thought I would try some nightingales….
Common Nightingale, Sotos de Albolafia, Córdoba, Andalucía, Spain.
In Case You Might Miss…
- 2024 Opinion-havers opine, two weeks out.
- Trump media blitz (Rogan; Carlson).
- Kamala and Biden’s decline (her view vs. the donor’s view).
- Boeing satellite explodes; Ortberg seeks culture change.
Politics
“So many of the social reactions that strike us as psychological are in fact a rational management of symbolic capital.” –Pierre Bourdieu, Classification Struggles
2024
Less tjhwo weeks to go!
Friday’s RCP Poll Averages:
Lambert here: Big Mo shifts toward Trump, this week, even in WI (that is, if you ignore the entire concept of margin of error). Of course, we on the outside might as well be examining the entrails of birds when we try to predict what will happen to the subset of voters (undecided; irregular) in a subset of states (swing), and the irregulars, especially, who will determine the outcome of the election but might as well be quantum foam, but presumably the campaign professionals have better data, and have the situation as under control as it can be MR SUBLIMINAL Fooled ya. Kidding!.
“Two Weeks to Go, but Only One Way to Stay Calm” [New York Times]. “‘Keep calm and throw it in the average’ is the best way for a voracious consumer of polling data to stay sane this election season…. To put this year’s post-Labor Day race into some historical polling context, I looked at how much one frequently cited polling average, that of RealClearPolitics, has moved going back through the 2008 election…. In 2008, shortly after Labor Day, John McCain actually held close to a three-point lead in national polls, but by Election Day, things had swung heavily toward Barack Obama, with the RealClearPolitics average showing Obama leading by around seven points — a 10-point range of results over the course of about two months…. . In 2020, a race in which Joe Biden consistently led in the national polls, the polling average still moved in a range from around Mr. Biden +6 to Mr. Biden +10. This year? Since Labor Day, the polls have moved from a high-water mark for Kamala Harris of +2.2 to a recent slim lead of just +0.9. So when we say the race is barely budging, it is barely budging,” And: “Trump’s very well-defined brand image anchors the election, leaving a very narrow band in which the race can trade.” I don’t think an election is a market, or a vote is a trade. More: ” I also think there’s the possibility that something methodological is behind the stability we see in the averages. Because some pollsters are weighting their surveys to a fairly fixed assumption about what the electorate will look like, including with regard to partisan makeup, it prevents numbers from moving as much as they might otherwise if the partisan makeup of their samples could float freely.” • Hmm.
* * * “James Carville: Three Reasons I’m Certain Kamala Harris Will Win” [New York Times]. “Ms. Harris will be elected the next president of the United States. Of this, I am certain. Here are three reasons: [1] Mr. Trump is a repeat electoral loser. This time will be no different… There simply do not seem to be enough voters — even in the battleground states — who turn out at Mr. Trump’s behest anymore when he’s simply preaching to his base. He has not learned from his electoral losses nor done the necessary work to assemble a broad electoral coalition in 2024. [2] Money matters, and Ms. Harris has it in droves….. With her field operation moving like a tremendous machine, it seems likely there has never been a greater disparity in voter contact efforts…. [3] It’s just a feeling. My final reason is 100 percent emotional. We are constantly told that America is too divided, too hopelessly stricken by tribalism, to grasp the stakes. That is plain wrong…. In two weeks, we not only have a chance to elect Kamala Harris as president, but a chance to bring finality to the sordid career of Donald Trump and drive MAGA into a prolonged remission.” • “Remission,” eh?
“Here’s What My Gut Says About the Election. But Don’t Trust Anyone’s Gut, Even Mine” [Nate Silver, New York Times]. “In an election where the seven battleground states are all polling within a percentage point or two, 50-50 is the only responsible forecast. Since the debate between Kamala Harris and Donald Trump, that is more or less exactly where my model has had it. Yet when I deliver this unsatisfying news, I inevitably get a question: ‘C’mon, Nate, what’s your gut say?’ So OK, I’ll tell you. My gut says Donald Trump. And my guess is that it is true for many anxious Democrats.” On the polling: “Instead [of shy Trump voters], the likely problem is what pollsters call nonresponse bias….. Trump supporters often have lower civic engagement and social trust, so they can be less inclined to complete a survey from a news organization. Pollsters are attempting to correct for this problem with increasingly aggressive data-massaging techniques, like weighing by educational attainment (college-educated voters are more likely to respond to surveys) or even by how people say they voted in the past. There’s no guarantee any of this will work. If Mr. Trump does beat his polling, there will have been at least one clear sign of it: Democrats no longer have a consistent edge in party identification — about as many people now identify as Republicans.” But: “Here’s another counterintuitive finding: It’s surprisingly likely that the election won’t be a photo finish…. With polling averages so close, even a small systematic polling error like the one the industry experienced in 2016 or 2020 could produce a comfortable Electoral College victory for Ms. Harris or Mr. Trump. According to my model, there’s about a 60 percent chance that one candidate will sweep at least six of seven battleground states…. Don’t be surprised if a relatively decisive win for one of the candidates is in the cards — or if there are bigger shifts from 2020 than most people’s guts might tell them.” • I guess we’ll see!
* * * “The electorate is changing. Here’s what that means for Trump and Harris” [Ron Brownstein, CNN]. “The groups that Harris needs to give her winning margins are the non-White and college-educated White voters (especially women in each case). The fact that both of those groups are increasing in the electorate, while Trump’s best cohort is shrinking, may give Harris a small swell in her sails. “The growing parts of the population are leaning Democrat, even in this time” when so many voters are discontented with conditions in the country, Frey said. The fact that women are likely to cast more ballots than men in all the swing states, he believes, will provide a small lift to Harris as well.” • Abortion + the war machine seems to be Harris’s view of a winning formula for college-educated White women… Commentary:
A rather important development: Harris appears to be slightly outperforming Biden 2020 among Trump's base of non-college White voters.
This is key because they make up a ton of the electorate, especially in MI, PA & WI. Explains why she's holding her own in MI, PA & WI. pic.twitter.com/ww9pnp3DxY
— (((Harry Enten))) (@ForecasterEnten) October 21, 2024
“Donald Trump Losing ‘Core Group’ Vital in 3 Battleground States” [Newsweek]. “Political analyst Harry Enten’s analysis shows Trump is leading nationally among noncollege educated white voters by 27 points, down from 31 points in 2020 and 33 points in 2016. ‘That might not seem like a lot, but given that we’re seeing these double digit gains among Black voters or among Hispanic voters in some of the polls, the fact that we’re seeing this core group of supporters actually moving away from him, not just off of the 2016 baseline, but the 2020 baseline as well, I think that’s a rather interesting development,’ Enten said… Enten said that the shift mattered because noncollege educated white voters make up 40 percent of the electorate nationally, but 51 percent of the electorate in the key battleground states of Pennsylvania, Michigan and Wisconsin…. ‘Donald Trump is making huge gains with groups that make up a smaller percentage of the electorate, but these groups that he’s losing a little bit of make up a much larger portion of the electorate,’ Enten explained. Enten’s analysis also revealed that Trump’s vote share among noncollege educated white voters is declining in Pennsylvania, Michigan and Wisconsin, with his lead currently standing at 19 points, compared to 22 points in 2020 and 25 points in 2016. ‘Trump is ahead, but his margins are smaller,’ Enten said. ‘And when you have a shrinking margin for Donald Trump, among his core group that makes up the majority of voters, it can make up for big shifts among smaller groups in the electorate and this is why Kamala Harris is still in the ball game right now.'” • Hmm.
“2024 Election Could Hinge on Tiny Shifts in the Electorate” [Ed Kilgore, New York Magazine]. ” This apparently very close presidential election reflects a deeply divided electorate where the potential changes in either direction we all talk about constantly are glacial and arguably self-canceling..,. Very big differences in the direction of the country will flow from tiny shifts in one direction or another of a closely divided electorate. It’s why anxiety levels are so high right now among those paying avid attention to politics, even though the outcome may depend on “low-propensity voters” barely paying attention at all.”
“Citizen Forecasting of the 2024 Presidential Election: Last Soundings” [Larry Sabato’s Crystal Ball]. “Asking people who they believe will win the election, as opposed to who they are going to vote for, can be an effective tool for trying to figure out who is favored in an election. A series of four different polls asking Americans who they believe will win the election showed that more expected Donald Trump to win during the summer, but more recently these expectations have moved in favor of Kamala Harris.” A Keynsian beauty contest! More: “A growing body of evidence indicates that ‘citizen forecasting‘ (CF), as the latter method has been called, makes for more accurate predictions of the winner. Indeed, studies of CF in the United States and the United Kingdom, as well as work on other democracies (such as Canada, France, or Germany) have demonstrated that voter expectations outperform voter intentions in terms of predictive accuracy, yielding a higher level of statistical and substantive significance. Inspired by this impressive track record, we have been systematically recording citizen forecasts with regard to the 2024 U.S. presidential election, via nationally representative samples of American voters, as drawn by the Verasight survey team.” And: “This current citizen forecast points to a Harris victory in November.” But: “Verasight polls conducted June 20-26, July 20-22, Aug. 20-26, Sept. 20-Oct. 2. N = 750 for all four polls.” • The last polling was still during Harris’s honeymoon period, three weeks ago. And that’s a long time in politics.
* * * “Will long COVID sway the 2024 election? These Rutgers researchers think it could” [USA Today]. “An aging U.S. population and the rise of long COVID mean voters with disabilities may have more of an impact on the upcoming election than ever before, a new Rutgers University report finds…. Between aging, long COVID and other factors, one in six eligible voters in the U.S. now has a disability of some type, the Rutgers researchers found. That’s double the growth rate of voters without disabilities, their report said…. Historically, these voters have splintered into smaller groups, each lobbying for its specific needs to deal with developmental disabilities, mobility challenges or other issues. But the various communities came together during the pandemic around imperatives like access to health care and employment, Schur said…. ‘Overall, there is no real partisan advantage. When there was a blue wave in 2018, people with disabilities were part of the blue wave. There wasn’t really a big difference, and I don’t expect a really big difference this year,’ [Douglas] Kruse said. ‘But it is true, as Lisa [Schur] says, that health care is more important to people with disabilities. And I think that tends to favor the Democrats.'” That might depend on what their experience with the health care system actually is. And: “One more fact about potential voters with disabilities heading to the polls across the country this year: There will be more women (21.6 million) than men (18.6 million). That breaks down into 411,300 men and 533,900 women in New Jersey. The group of adults affected swells to 72.7 million — one in three eligible voters — after adding those who live with someone with a disability. Thought about that way, the electorate with disabilities now surpasses the Hispanic/Latino and Black voting demographics in the U.S., Kruse and Schur noted.”
* * * Kamala (D): “The Clock Is Ticking on Kamala Harris” [Politico]. “The evening’s moderator, Wisconsin conservative talk show host turned anti-Trump writer Charlie Sykes, opened with the political equivalent of a fastball down the middle of the plate. Perched beneath a ‘Country Over Party sign,’ Sykes asked Harris for her pitch to the voter who supported Republicans from yesteryear but are uneasy now about casting a ballot for a Democrat. The vice president began by citing ‘the lived experience’ of most Americans — sounding more like a graduate student from down the road in Madison than most Americans — of having much in common. She repeated a line from her stump speech about how Americans ‘love our country’ before praising democracy, the rule of law and the Constitution. Then, making a little progress, she invoked her service on the Senate Intelligence Committee, where lawmakers from both parties put aside partisanship to focus on protecting the ‘security and well-being’ of all Americans. That, Harris concluded, ‘is at stake.’ That was it. Harris said nothing specific about how she’d govern, mentioned no looming issue on which she’d work with Republicans and offered no reassurances about leading the country from the political center. And, of course, there was no critique of her own party or even an expression of sympathy or understanding about why voting for a liberal could be difficult for a longtime conservative. There wasn’t even a reference to her previous commitments to include a Republican in the Cabinet or create a bipartisan council of advisers. And this was in response to the opening question from a pre-selected moderator who is supporting her campaign!” • Everything about this is bad: the candidate, the staffwork, and the net effect on those invested in her, like Sykes, who might well feel they’d been played.
Hallie Jackson asked Harris yesterday: "Can you say that you were honest with the American people about what you saw in those moments with President Biden, as you were with him again and again repeatedly in that time?"
Harris: "Of course. Joe Biden is an extremely accomplished,… pic.twitter.com/HMykeLm5MD
— Alex Thompson (@AlexThomp) October 23, 2024
What others saw:
At a Chevy Chase fundraiser in June 2023, Bill Reichblum tells Woodward of Biden: “He never completed a sentence….He told the same story three times in exactly the same way and it meandered so much…Frankly, my impression was there were times…it was as though we didn’t exist.… pic.twitter.com/Vav7clSMGQ
— Alex Thompson (@AlexThomp) October 23, 2024
I suppose a Democrat loyalist would say “She has to say that,” but it sticks in my craw. And Biden is also, we may forget, still President. Why is that OK?
Kamala (D): “Detroit Muslim leader ejected from Kamala Harris rally, deepening rift between Democrats and Arab Americans” [Detroit Metro Times]. “Ahmed Ghanim, a Democrat, says he accepted an invitation to the event and was seated in the Royal Oak Music Theatre when a campaign organizer ordered him to leave. ‘She took me to the door, and she closed it, and I found two police officers waiting there, and she said, ‘You have to leave right now,” Ghanim tells Metro Times. ‘I asked why she was kicking me out. She wouldn’t answer. I was very calmly asking why I was being kicked out.’ He continues, ‘I was just wearing a suit and a white shirt. I said, ‘OK,’ and I left. The police officer said, ‘You either leave now or I’ll put you in the back of my car.'” Nice. The Harris campaign statementL “The campaign was swiftly informed by Emgage Action [Ghanim’s organizaiton] of yesterday’s incident and looked into it,’ the statement reads, referring to a Muslim American advocacy group. ‘Our campaign regrets this action and its impact on Dr. Ghanim and the community, and he is welcome at future events. We value our relationship with the Muslim American community and are committed to ensuring all community and political spaces are welcoming and respectful to every American.'” • Terrible staffwork, both in Ghanim’s ejection and the statement (in which the word “apologize” does not appear). Despite the statements blah blah blah, it looks to me like the Harris campaign already had the cops wired up, and had a list (with photos) of attendees to bar.
Kamala (D): “What Kamala Harris Needs to Do to Win Over More Black Men” [New York Magazine]. “Democrats have known since Hillary Clinton’s loss in 2016 that carrying the all-important industrial midwestern states requires maximum turnout in the Black communities of Detroit, Milwaukee, Pittsburgh, and Philadelphia. The problem is that Dems have assumed they could generate Obama-era levels of excitement for Hillary Clinton and Joe Biden — and now Kamala Harris — without a substantial, sustained political investment up front.” And: “‘You’re going to pick on Black men and blame us for everything. What did you deliver to Black men in the past four years?’ the activist and commentator Van Jones said at a recent university discussion in California. ‘We wanted the George Floyd police-reform act. Didn’t get it. We wanted the John Lewis Voting Rights Act. Didn’t get it. And we wanted the EQUAL Act, the next step in criminal justice reform, and didn’t get that. Three things we wanted, and we got zero, zilch, nunca, nada, nothing.’ By contrast, Jones noted, Trump signed the First Step Act, which granted early release from federal prison to more than 30,000 people — a monumental rollback of mass incarceration that Democrats rarely discuss.” • Hmm.
Kamala (D):
NEWS
Vice President Kamala Harris is planning to deliver her closing argument Tuesday in a speech on the National Mall.
Sources tell the Washington Post that Harris’ speech will talk about January 6 but will focus more broadly on her thoughts on Trump’s threat to democracy.… pic.twitter.com/FVM52Jl01q
— Yashar Ali 🐘 (@yashar) October 23, 2024
Chutzpah. Even Obama 2012 didn’t do that. I grant that the setting tops Carlson and Rogan.
Kamala (D): Biden on the trail:
Biden in NH lists what Trump would do as president and says: “we gotta lock him up” and then, appearing to realize how his comments would be taken, adds: “politically, lock him up.” pic.twitter.com/cw3X8RzKn2
— Alex Thompson (@AlexThomp) October 22, 2024
Thanks, Joe. We knew what you meant.
* * * Here we go! 🇺🇸 @realDonaldTrump pic.twitter.com/bgja6JPqsQ
— Joe Rogan Podcast (@joeroganhq) October 22, 2024
JRE = Joe Rogan Experience.
Trump (R): Media blitz (2):
Our live tour is not over yet. For the grand finale, we’ll be in Glendale, AZ, on October 31st with special guest President Donald J. Trump. All profits will be donated to hurricane relief efforts. We hope to see you there.
Get tickets to our biggest show yet at… pic.twitter.com/00756VvITT
— Tucker Carlson (@TuckerCarlson) October 23, 2024
* * * Trump (R) (Willis/McAfee): “Special prosecutor in Georgia’s case against Trump met with Biden officials” [Washington Times]. “Nathan Wade, who led the Fulton County election interference case against Donald Trump while having an affair with District Attorney Fani Willis, admitted to congressional investigators he met with White House officials several times but claimed he couldn’t remember the details…. Ms. Willis hired Mr. Wade even though he was a divorce lawyer with little experience prosecuting criminal cases. In 2022, he was paid nearly $700,000 to oversee the case against the former president, according to a co-defendant seeking to have the charges dismissed….. Mr. Wade acknowledged the Biden administration’s involvement in the case during the interview that was conducted on Oct. 15 by committee investigators. He verified the existence of invoices he submitted in which he billed for a ‘Conf. with White House counsel’ in Athens, Georgia, on May 23, 2022. When asked about each of the invoices obtained by investigators, Mr. Wade responded 58 times that he couldn’t remember details.” • What reason could the White House possibly have for getting involved in a state prosectution? Seems odd.
* * * “Elon Musk ‘Jokes’ in a Church About Someone Killing Kamala Harris” [Rolling Stone]. “Elon Musk keeps trying to stick his ‘joke’ about somebody assassinating Vice President Kamala Harris. Over the weekend, Musk remarked, again, that ‘no one’s even bothering to try to kill Kamala’ … Acting as a Donald Trump surrogate — in addition to the $75 million moneyman behind a leading Trump Super PAC — Musk spoke Saturday night at the Life Center megachurch in Harrisburg, Pennsylvania, which is notable for launching the career of Christian supremacist God-rocker Sean Feucht.” • I wouldn’t be surprised if Musk’s involvement, at all levels, didn’t turn out to be a net negative. He’s a loose cannon, and the Trump campaign doesn’t need two. (I’d like a lot less Musk, and a lot more Kennedy. I wouldn’t call Kennedy a loose cannon, so much as his own cannon. Apparently, a Townall with Trump, Kennedy, and Tulsi was cancelled; unfortunate, in my view).
* * * AZ: Impressive:
Arizona Dem running against Kari Lake foe the US Senate, RUBEN GALLEGO, had promised to visit all 22 of the state's federally recognized tribes. But there was no road leading into the Havasupai village of 639 people. So he trekked in on foot. It took him 4 hours to walk there.
— Lise Horton Is Writing (@lisekimhorton) October 19, 2024
Perhaps a stunt, but still impressive.
GA: “In battleground Georgia, poor people see no reason to vote. That decision could sway election” [Associated Press (KLG)]. “Located about 80 miles (130 kilometers) south of Atlanta, Bibb County is the kind of place where Vice President Kamala Harris would need to run up her margin in order to defeat Donald Trump in this year’s election, a strategy that helped Biden win the state four years ago as he promised to lift up Black Americans. It won’t be easy: Bibb County never recovered all the jobs lost during the pandemic, and Labor Department data show it had more jobs in 2019 under Trump than it does now. Trump, the former president, sees himself as having an opportunity with Black voters, particularly men. But he and Harris have one thing in common: Each will have a difficult time persuading people to turn out who typically sit out elections. More than 47,000 people in Bibb County were eligible to vote in 2020 and didn’t, a figure roughly four times Biden’s margin of victory across the entire state.” But: “But the more nonurban parts of Georgia are only part of the electoral puzzle. It’s a dramatically different story in Atlanta and its vote-rich suburbs where enthusiasm runs high for both Harris and Trump, although often divided by race.” However: “Nonvoters have basic, urgent needs the campaigns don’t address.”
NV: “The early voting blog, 2024” [John Ralston (ick), Nevada Independent]. “Rurals matter. Especially when they are turning out 3.5 points above their registration and producing landslide ballot wins (58-20) over the Ds….. Dems are only winning the urban Nevada ballot race by 1 percent – 38-37. Repubs have a nearly 3 percent turnout advantage statewide.” • Interesting, but early days yet.
NV: “As Harris Courts Sun Belt, Housing Costs Stand in Her Way” [New York Times]. “In a state that relies on hourly wage workers in tourism and service jobs, many cannot find an affordable place to live… Many working-class voters say a promise has been broken, and they are looking for someone to blame… Many once-reliable Democrats say the issue has eroded their trust in politicians. In the state’s Democratic hub, that means turning away from Vice President Kamala Harris. ‘When we got the new president, I didn’t hear nothing, I didn’t see any changes,’ Maria Ocampo, 54, who has voted Democratic for decades, said of the Biden administration. This year, she does not plan to vote at all.” • Innocent bystander: “Wasn’t Lina Khan working on price-gouging landlords?” Harris Campaign: “Who?”
Realignment and Legitimacy
“Early-voting data shows Republican reversal appears to be paying off” [WaPo] “Democrats have embraced early voting for years, in part because it leaves less to chance on Election Day, when busy schedules, bad weather or illness can keep voters from making it to the polls. Known as “banking” votes, early voting also allows campaigns to be more efficient with resources as Election Day draws near by focusing attention on a smaller and smaller group of voters who have not yet cast ballots.” • Another way of saying this is that early voting skews toward partisans who don’t actually need to study what the candidates are saying. Why we are allowing that population, and the convenience of party operatives, to drive how our elections are run is an open question.
Syndemics
“I am in earnest — I will not equivocate — I will not excuse — I will not retreat a single inch — AND I WILL BE HEARD.” –William Lloyd Garrison
Covid Resources, United States (National): Transmission (CDC); Wastewater (CDC, Biobot; includes many counties; Wastewater Scan, includes drilldown by zip); Variants (CDC; Walgreens); “Iowa COVID-19 Tracker” (in IA, but national data). “Infection Control, Emergency Management, Safety, and General Thoughts” (especially on hospitalization by city).
Lambert here: Readers, thanks for the collective effort. To update any entry, do feel free to contact me at the address given with the plants. Please put “COVID” in the subject line. Thank you!
Resources, United States (Local): AK (dashboard); AL (dashboard); AR (dashboard); AZ (dashboard); CA (dashboard; Marin, dashboard; Stanford, wastewater; Oakland, wastewater); CO (dashboard; wastewater); CT (dashboard); DE (dashboard); FL (wastewater); GA (wastewater); HI (dashboard); IA (wastewater reports); ID (dashboard, Boise; dashboard, wastewater, Central Idaho; wastewater, Coeur d’Alene; dashboard, Spokane County); IL (wastewater); IN (dashboard); KS (dashboard; wastewater, Lawrence); KY (dashboard, Louisville); LA (dashboard); MA (wastewater); MD (dashboard); ME (dashboard); MI (wastewater; wastewater); MN (dashboard); MO (wastewater); MS (dashboard); MT (dashboard); NC (dashboard); ND (dashboard; wastewater); NE (dashboard); NH (wastewater); NJ (dashboard); NM (dashboard); NV (dashboard; wastewater, Southern NV); NY (dashboard); OH (dashboard); OK (dashboard); OR (dashboard); PA (dashboard); RI (dashboard); SC (dashboard); SD (dashboard); TN (dashboard); TX (dashboard); UT (wastewater); VA (wastewater); VT (dashboard); WA (dashboard; dashboard); WI (wastewater); WV (wastewater); WY (wastewater).
Resources, Canada (National): Wastewater (Government of Canada).
Resources, Canada (Provincial): ON (wastewater); QC (les eaux usées); BC (wastewater); BC, Vancouver (wastewater).
Hat tips to helpful readers: Alexis, anon (2), Art_DogCT, B24S, CanCyn, ChiGal, Chuck L, Festoonic, FM, FreeMarketApologist (4), Gumbo, hop2it, JB, JEHR, JF, JL Joe, John, JM (10), JustAnotherVolunteer, JW, KatieBird, KF, KidDoc, LL, Michael King, KF, LaRuse, mrsyk, MT, MT_Wild, otisyves, Petal (6), RK (2), RL, RM, Rod, square coats (11), tennesseewaltzer, Tom B., Utah, Bob White (3).
Stay safe out there!
Airborne Transmission: Covid
“Transmission of SARS-CoV-2 on aircraft: A scoping review” (preprint) [medRxiv]. From the Abstract: “This review summarises reported contact-tracing data and evaluates the secondary attack rates (SAR) and factors associated with SARS-CoV-2 transmission in aircraft, to provide insight for future decision making in the context of future respiratory pandemics…. Our results are consistent with sporadic clusters happening onboard aircraft. Close proximity to COVID-19 cases within the aircraft was associated with a higher [secondary attack rates (SAR)].” • Sitting closer to the aisle and moving about the cabin is also associated with greater risk. In addition, transmission will also depend on the ventilation patterns in any given aircraft.
Infection: Covid
“Incident COVID-19 infections before Omicron in the US” (preprint) [medRxiv]. From the Abstract: “[W]e retrospectively estimate daily incident infections for each U.S. state prior to Omicron. To this end, reported COVID-19 cases are deconvolved to their date of infection onset using delay distributions estimated from the CDC line list. Then, a novel serology-driven model is used to scale these deconvolved cases to account for the unreported infections. The resulting infections incorporate variant-specific incubation periods, reinfections, and waning antigenic immunity. They clearly demonstrate that the reported cases fail to reflect the full extent of disease burden in all states. Most notably, infections were severely underreported during the Delta wave, with an estimated reporting rate as low as 6.3% in New Jersey, 7.3% in Maryland, and 8.4% in Nevada. Moreover, in 44 states, fewer than 1/3 of infections appear as cases reports. Therefore, while reported cases offer a convenient proxy of disease burden, they fail to capture the full extent of infections, and can severely underestimate the true disease burden.” Here is a summary of the method: “First, we estimate the delays from positive specimen to report date and use them to push back the reported cases to their sample collection dates. Next, we estimate the delay from symptom onset to sample collection, combine this with variant-specific infection-to-symptom delays, and use these to push back the cases to infection onset. The resulting case estimates are aggregated across variant categories and adjusted by the case ascertainment ratio, estimated with seroprevalence survey data and a model for antigenic immunity.” This is above my paygrade. Perhaps an epidemiologist in the readership can comment.
Elite Maleficence
Still killing people:
WRONG WRONG WRONG WRONG WRONG WRONG WRONG WRONG https://t.co/uP4sCT0VCy
— Nate Bear (@NateB_Panic) October 22, 2024
Wastewater | |
This week[1] CDC October 14 | Last Week[2] CDC (until next week): |
|
|
Variants [3] CDC October 12 | Emergency Room Visits[4] CDC October 12 |
|
|
Hospitalization | |
★ New York[5] New York State, data October 22: | National [6] CDC September 28: |
|
|
Positivity | |
National[7] Walgreens October 21: | Ohio[8] Cleveland Clinic October 19: |
|
|
Travelers Data | |
Positivity[9] CDC September 30: | Variants[10] CDC September 30: |
|
|
Deaths | |
Weekly Deaths vs. % Positivity [11] CDC October 12: | Weekly Deaths vs. ED Visits [12] CDC October 12: |
|
|
LEGEND
1) ★ for charts new today; all others are not updated.
2) For a full-size/full-resolution image, Command-click (MacOS) or right-click (Windows) on the chart thumbnail and “open image in new tab.”
NOTES
[1] (CDC) Good news!
[2] (CDC) Last week’s wastewater map.
[3] (CDC Variants) KP.* very popular. XEC has entered the chat.
[4] (ED) Down.
[5] (Hospitalization: NY) Steadily down.
[6] (Hospitalization: CDC). I see the “everything in greenish pastels” crowd has gotten to this chart.
[7] (Walgreens) A pause.
[8] (Cleveland) Dropping.
[9] (Travelers: Positivity) Down.
[10] (Travelers: Variants). No XEC.
[11] Deaths low, positivity down.
[12] Deaths low, ED down.
Stats Watch
There are no official statistics of interest today.
Manufacturing: “Boeing’s bad year just keeps getting worse: One of its satellites has exploded in orbit, with debris becoming a potential threat to other satellites” [Fortune]. “A Boeing-made communications satellite has exploded, with debris floating that could potentially be a threat to other satellites at some point. Intelsat, the owner of the satellite, is reporting “the total loss” of the device in an update on its Website. The company is working with Boeing and government agencies to determine the cause of the mishap…. The U.S. Space Force says it is tracking 20 pieces of debris from the destroyed satellite and there was no immediate threat to other orbiting space equipment. Other observers, though, have seen higher amounts of debris. ExoAnalytic Solutions told SpaceNews it was tracking 57 pieces of debris and was warning operators of spacecraft that could be at risk of collision. And Russia’s space agency said it was tracking more than 80 fragments.” • Oopsie.
Manufacturing: “Boeing reports $6 billion quarterly loss as striking workers vote whether to accept contract offer” [Associated Press]. ” Boeing reported a third-quarter loss of more than $6 billion before turning its attention to whether striking factory workers would accept a contract offer Wednesday and end a walkout that has crippled the company’s airplane production for nearly six weeks…. Boeing hasn’t had a profitable year since 2018, and Wednesday’s numbers represented the second-worst quarter in the manufacturer’s history.The company burned nearly $2 billion in cash, in the quarter, weakening its balance sheet, which is loaded down with $58 billion in debt. Chief Financial Officer Brian West said the company will burn cash through 2025, but at a slower pace.”
Manufacturing: “Boeing’s New C.E.O. Calls for ‘Culture Change’ as Strike Vote Looms” [New York Times]. “In a message to employees, Mr. Ortberg shared a speech that he planned to deliver to investor analysts on a call later in the day to discuss Boeing’s quarterly financial results. In it, he offered a diagnosis: The company had lost too much trust, gained too much debt and made too many mistakes. To put Boeing back on the right path would require ‘fundamental culture change,’ stabilizing the business and improving execution. ‘Our leaders, from me on down, need to be closely integrated with our business and the people who are doing the design and production of our products,’ he said. ‘We need to be on the factory floors, in the back shops and in our engineering labs. We need to know what’s going on, not only with our products, but with our people.'” • Fine. Restore defined-benefit pensions (and put a union member on the Board, as I keep screaming). Here are Ortberg’s complete remarks.
Manufacturing: “Intelsat 33e breaks up in geostationary orbit” [Space News]. “An Intelsat spokesperson said the satellite was not insured at the time of the issue.” • Surely not wise, with a Boeing product?
Today’s Fear & Greed Index: 71 Greed (previous close: 73 Extreme Greed) [CNN]. One week ago: 66 (Greed). (0 is Extreme Fear; 100 is Extreme Greed). Last updated Oct 22 at 2:37:54 PM ET.
Gallery
One of my favorite flowers:
Monet, Irises pic.twitter.com/ElVphj6eDM
— Impressions (@impression_ists) October 20, 2024
“The Tech Coup: A New Book Shows How the Unchecked Power of Companies Is Destabilizing Governance” (interview) [Stanford University]. Interview with Stanford institute for Human-Centered AI Policy Fellow Marietje Schaake. “In what ways are private companies increasingly taking on functions normally assumed by states? In the digital realm, companies’ control of information, unfettered agency, and power to act have almost overtaken that of governments. For example, in the private intelligence sector, companies like NSO Group Technologies with its Pegasus spyware products are creating and selling the capability to hack into people’s devices. This means that anyone with the financial resources to purchase Pegasus spyware can access the capabilities of intelligence services and hack into the very private information of political opponents, judges, journalists, critical employees, competitors, and others. Another striking example is that of offensive cyber capabilities. In the name of defending their clients or their networks, companies are attacking hackers across borders, using ‘offense as defense.’ And notice that I’m talking not only about big tech companies but also small ones, because there’s de facto power that comes from the development of digital technologies.”
“How Your Brain Detects Patterns without Conscious Thought” [Scientific American]. “In an experimental trial, participants viewed a series of the face images…. During the experiment, neurons in each participant’s hippocampus and entorhinal cortex gradually began to respond not only to the face being presented but also to faces directly connected to it on the triangle. When asked whether they noticed any pattern in the order of the images, the participants said they didn’t. But their brain cells still learnt the pattern, showing that the brain can recognize patterns without conscious awareness. In the breaks between trials, the participants’ ‘face’ neurons replayed what they had learnt, cycling through the patterns on their own without being stimulated to do so. ‘This is something that is not explicit, it is implicit. And the brain gets it, essentially, very quickly, and we can see those changes in the individual cells,’ says Fried.” • Patterns generally, or optimized for faces?
For our numismatists:
Distribution of Roman Coins Found. pic.twitter.com/XC9X2E5jDR
— Illegitimate Scholar🧭 (@ill_Scholar) October 7, 2024
Contact information for plants: Readers, feel free to contact me at lambert [UNDERSCORE] strether [DOT] corrente [AT] yahoo [DOT] com, to (a) find out how to send me a check if you are allergic to PayPal and (b) to find out how to send me images of plants. Vegetables are fine! Fungi, lichen, and coral are deemed to be honorary plants! If you want your handle to appear as a credit, please place it at the start of your mail in parentheses: (thus). Otherwise, I will anonymize by using your initials. See the previous Water Cooler (with plant) here. From SC:
SC writes:
For more than a decade, I’ve been intrigued by the genus Tithonia, aka “Mexican Sunflower.” I first encountered it back in the ’00s or early ’10s when I read that it was used as green manure in parts of Africa, planted along the edges of fields and harvested for use as Nitrogen rich soil amendment. At first, I thought that it was fixing atmospheric Nitrogen but I later learned that it merely accumulates already-present soil Nitrogen into its tissues. It’s a cheery, attractive plant, sort of a giant puff-ball of orange daisy-like blossoms. The blossoms are well-suited to the feeding anatomy of butterflies and hummingbirds. Starting in the mid-to-late ’10s, I have been annually providing a bunch of these to a local social services agency that plants them outside its meeting rooms. The plants attract hummingbirds, which provide a morale boost to staff and clients. At, I think, clientele initiative, the agency subsequently started a horticulture sub-agenda in one of its programs, an on-site day partial care program for mentally-ill homeless people, and that has branched out into veggie, decorative and herb gardening at the agency site.
A few years ago, a sibling planted two of these (of species T. Rotundifolia, that reputedly grows to 6′ height) and aggressively watered them. They grew to the eaves of her house, 8′ at least, and were so leafy and dense that you couldn’t see through them. The plants had hundreds of blossoms and lots of hiding places, a kind of hummingbird heaven. Such a large plant from a small seed.
This photo is of an example of T. speciosa (in my experience, less tall and bushy than rotundifolia), that reputedly reaches 4-5′ height. A friend who regularly accepts plants from my “backyard nursery” passed it further on. It was started from seed in mid-Winter 2024. The recipient evidently aggressively watered and fertilized it; the plant towers at 9′. I wonder what the neighbors make of it.
Wow. I could really file this under “Look for the helpers,” too!
Readers: Water Cooler is a standalone entity not covered by the annual NC fundraiser. Material here is Lambert’s, and does not express the views of the Naked Capitalism site. If you see a link you especially like, or an item you wouldn’t see anywhere else, please do not hesitate to express your appreciation in tangible form. Remember, a tip jar is for tipping! Regular positive feedback both makes me feel good and lets me know I’m on the right track with coverage. When I get no donations for three or four days I get worried. More tangibly, a constant trickle of donations helps me with expenses, and I factor in that trickle when setting fundraising goals:
Here is the screen that will appear, which I have helpfully annotated:
If you hate PayPal, you can email me at lambert [UNDERSCORE] strether [DOT] corrente [AT] yahoo [DOT] com, and I will give you directions on how to send a check. Thank you!